To Detect Evasive CEOs, Use AI
Dodgy answers in earnings calls can be predictive of a company’s near-term financial performance
Based on the research of Andrew Whinston
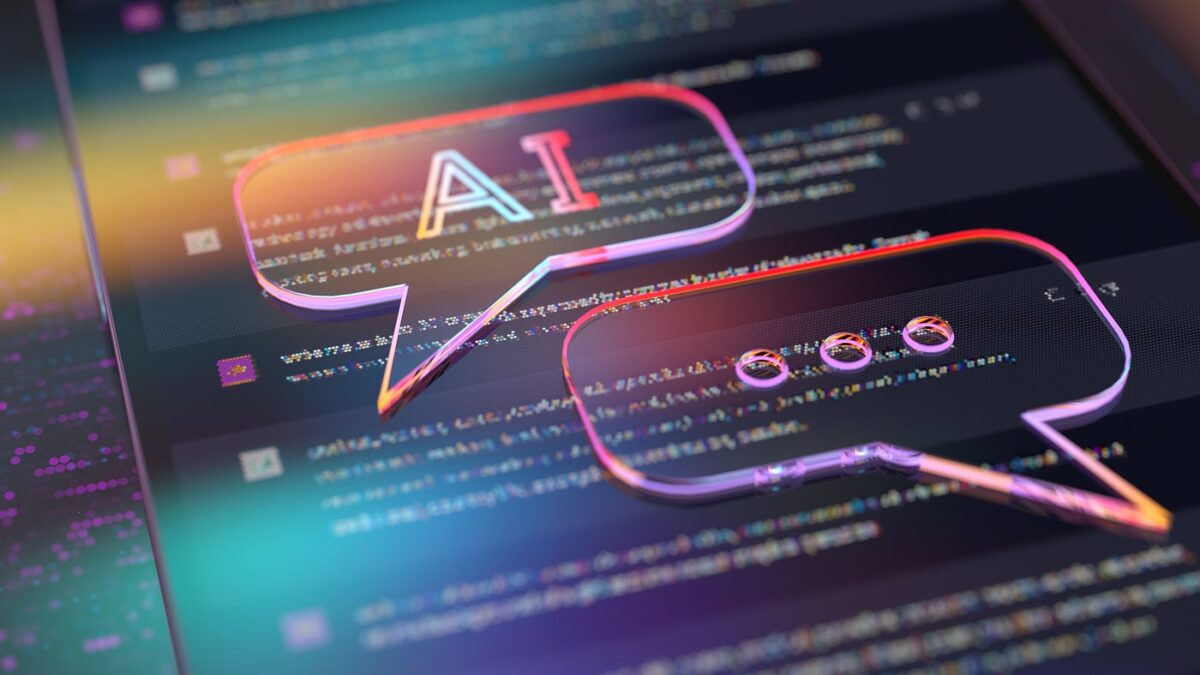
After each quarter, many public companies host formal conference calls. Executives present market analysts with an overview of recent financial performance and then take questions.
Unlike the presentations, these Q&A sessions are unscripted. If analysts seek negative information, company leaders have been known to give enigmatic answers as they dance around the truth.
Such squishiness can be measured using artificial intelligence tools, finds new research from Andrew Whinston, a professor of information, risk, and operations management at Texas McCombs. What’s more, such tools can help investors predict a company’s near-term financial performance.
Whinston guided the research of two McCombs graduate students — Yanzhen Chen, now of the Hong Kong University of Science and Technology, and Huaxia Rui, now of the University of Rochester. They designed and trained two algorithms to detect evasiveness and incoherence in human language.
For evasiveness, the algorithms looked for dissimilar topics between questions and answers. For incoherence, they examined the flow of replies. They quantified both characteristics in answers given by the leaders of S&P 500 companies during 6,802 earnings calls between 2006 and 2018.
“It’s hard for a person to sit there listening to people talk all day, and these machines are systematic and process information rapidly,” says Whinston, who also is the Hugh Roy Cullen Centennial Chair in Business Administration and director of the Center for Research on Electronic Commerce at The University of Texas at Austin.
On the average call, the researchers found high levels of both evasiveness — 60% of the possible maximum — and incoherence — 62% of the max.
Incoherence Docks Earnings
Next, they compared levels of evasiveness and incoherence to the companies’ financial performance. Controlling for a variety of other factors, they found that incoherence affected both next-day stock prices and next-quarter earnings.
- A 40 percentage-point rise in incoherence level corresponded to a 0.74% drop in the 1-day return.
- It also corresponded to next-quarter earnings that were 5.6 cents per share less than forecast by analysts, per unit of seasonal earnings change volatility.
Evasiveness had a weaker effect, they found. It helped predict lower earnings but not returns.
“Clearly, managers’ failure to answer coherently during earnings calls is negatively perceived by investors,” Whinston says.
Machine learning and natural language processing, the two AI tools the team employed, have evolved rapidly since the research concluded. But Chen, the lead graduate student, says its models remain viable. Smaller market players, in particular, could benefit from using similar tools.
“Non-institutional investors should indeed utilize these tools,” Chen says. “Failing to do so could mean missing out on critical information that could impact investment decisions and potentially place them at a disadvantage.”
In the long run, the researchers suggest, such AI tools might help job interviewers evaluate applicants and voters evaluate candidates.
For now, however, they hope these tools will level the playing field between investors and executives. They write, “In this cat-and-mouse game of information seeking, machines may ultimately win, leaving only one viable option for managers: be honest and be forthright.”
“Conversation Analytics: Can Machines Read Between the Lines in Real-Time Strategic Conversations?” is published online in Information Systems Research.
Story by Kiah Collier
About this Post
Share: