To Remove Corporate Bias, Let Algorithms Summarize Earnings
New research finds that algorithm-created corporate earnings summaries offer benefits over management-penned releases
Based on the research of Brian White
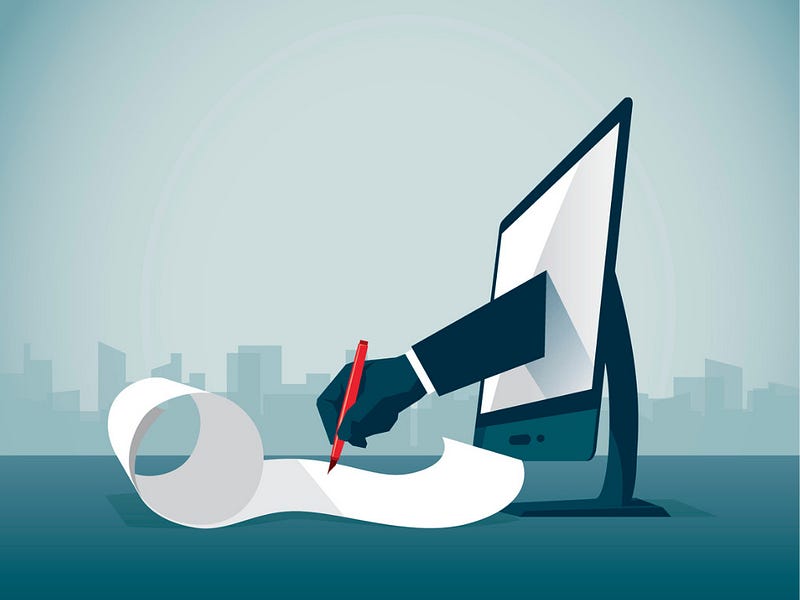
Corporate earnings releases are critical to investors’ decisions to buy and sell stock. But do investors react differently when those releases are written by a computer?
Earnings releases created by algorithms use statistical techniques to identify the most important sentences in a disclosure, which are then used to form the summary. Such summaries limit human bias while capturing all of the important information, according to Brian White, associate professor of accounting at Texas McCombs. They also make investors more confident in their decisions.
In a new paper, White and his co-authors — Eddy Cardinaels from Tilburg University and Stephan Hollander from KU Leuven — compared investor behavior when presented with algorithm-based summarization of corporate disclosures, specifically earnings releases, to summaries written by management teams. White talked about the research and its potential impact.
What prompted your research interest in corporate reports?
Companies are disclosing more information than ever before. Some of that is mandatory, a lot is voluntary. Companies increasingly issue summaries of their disclosures — earnings releases are a prominent example. It’s not surprising that when you have a lot of disclosures, you would feel inclined to issue a summary because people have limited attention. A summary makes the information more digestible for people.
Firms have incentives and the discretion to potentially bias those summaries. So, compared to the underlying text they’re summarizing, firm managers might cherry-pick good news and information that shows their firm in the best light.
That got us wondering if there might be a role for what we call automatic summarization or automatic algorithm-based summarization. It could provide useful summary information — playing the role firms want — but with less bias, because they’re relatively neutral.
How did you analyze corporate summaries? What did you learn?
We looked at earnings releases because they tend to be one of the most prominent, frequent, and influential disclosures.
We asked an independent expert to come up with a summary of a couple of earnings releases. Then we compared our automatic summaries and the actual firm’s management summary to the expert summary. We found, pretty surprisingly, that the automatic summary was more similar to the expert summary than the firm management summary was to the expert summary. That tells us a couple of things. First, these algorithms are doing a good job of summarizing in the same way as an expert. It also tells us that firm management is doing something different: They’re giving a summary that’s much more positive than the independent expert or the algorithm.
Then, we did a controlled laboratory experiment to test the effect of these different summary types on individual investor’s judgments: Participants provided estimates of equity value, the potential for future earnings growth, the favorability of the earnings release, and risk. Results for each of these measures pointed in the same direction. In other words, they reacted more favorably to the positively biased management summary. We found that summaries did matter.
Study participants did not know if the summaries were written by an algorithm or management. How did they react to each type?
Investors seem to buy into management’s positive spin in the summary. When they saw the management’s summary, they reacted more positively to the information. When they saw the automatic summary, they reacted less positively. On average, it’s no different than how investors would react if they saw the earnings release with no summary. But, pretty important, we still see a benefit of the automatic summary: We also measured their confidence in their judgments to take any action, and they were more confident when they got the earnings release with the automatic summary than with no summary.
Why is that?
We don’t have any direct evidence regarding why automatic summaries made them more confident, but I would speculate that it’s because the summaries were a better match for the underlying disclosure. Observing that match likely gives them more confidence in their conclusions.
Based on your research, what’s next for automatic summaries?
Overall, we were left feeling positive about the potential for these algorithm-based summaries to provide information that’s as useful — without the positive bias that’s being injected — as management summaries.
The Securities and Exchange Commission, in particular, has expressed interest in summarization methods. The SEC is aware that these summaries are a double-edged sword when they’re prepared by management. They’re useful, but they can also be biased. We want to provide some initial evidence that automatic summaries are potentially fruitful as an alternative. We’re fairly careful in the paper not to say, “We should be instituting automatic summaries for all firms now,” but they look promising.
“Automatic Summarization of Earnings Releases: Attributes and Effects on Investors’ Judgements” published online in Review of Accounting Studies.
Story by Sloan Wyatt
About this Post
Share: