Fighting Bias in Machine Learning
Improving fairness in the life-changing decisions made with algorithms
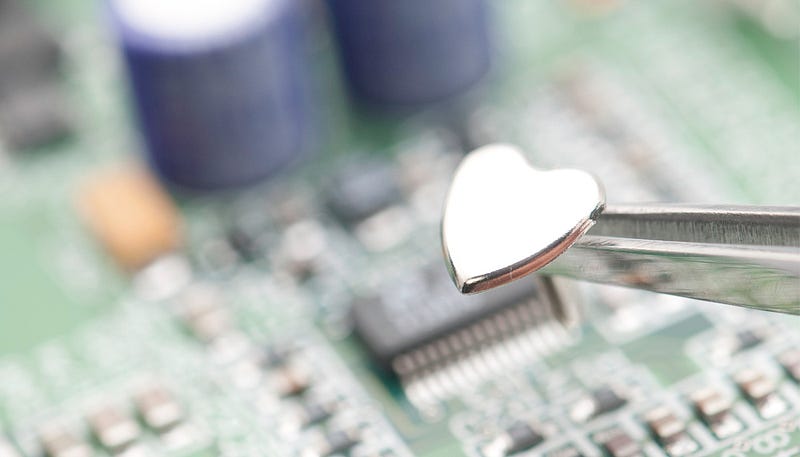
Technology can help us make better decisions. But it can also deepen existing inequalities.
Maria De-Arteaga, IROM assistant professor at Texas McCombs, studies the dangers of bias in machine learning, where an algorithm learns from patterns to make often critical, life-changing decisions.
De-Arteaga addresses how we can improve machine learning decisions, and she has researched challenges as diverse as coma patient recovery and where police are deployed to address neighborhood crime. She studied math at Universidad Nacional de Colombia in Bogota while working as an investigative journalist, then earned a doctorate from Carnegie Mellon University before joining the McCombs faculty in July 2020. De-Arteaga has been recognized for her work, most recently as a recipient of Google’s Award for Inclusion Research in 2020. We recently spoke about what prompted her interest in improving machine learning and the types of bias her research addresses.
What questions drive your machine learning research?
I focus on the risks and opportunities of using machine learning to support experts’ decisions. Part of that is characterizing bias: Where does bias come from, what types of biases are embedded in the data, and what are the risks of learning from this data? When you’re using machine learning to support decision making, you’re often giving recommendations in high-stakes settings, and you’re not making an autonomous decision. Part of my work has also looked at the role of humans in the loop.
The third part of my work is designing machine learning algorithms that can lead to better expert decision making, both in terms of overall quality and fairness.
Did any personal experience encourage your research focus?
At the beginning of my Ph.D. in machine learning and public policy, I was mostly focused on developing algorithms to address questions motivated by policy contexts. One example, in health care, I used data from EEG brain signals to predict whether a patient would recover from a coma. In an entirely different context, we looked at identifying patterns of sexual violence in El Salvador. Throughout all of these experiences, we would develop a solution and get results that were encouraging. But when we thought about potentially deploying the solution, the risk of overburdening or underserving a population kept surfacing as a big challenge. That’s what led me to refocus my attention on better understanding what the risks are and how can we address them.
For instance, in El Salvador emerging patterns of sexual violence could inform sexual education policies, but there was a risk: You’re obviously not going to identify patterns for those people who are not reporting — and people who are not reporting sexual violence are often not reporting it because they don’t trust the authorities who collect the data. Their lack of trust is, in many cases, warranted by their experiences with these institutions. So, you have this risk of a well-intentioned policy that ends up underserving a community that has already been historically marginalized.
More recently, you’ve examined risks and bias in predictive policing. What did you find?
Previously, researchers studied what happens when you use data from arrests and found that it can perpetuate bias in predictive policing practices. We studied the risk of bias when the data comes from victim reports. We looked at data from Bogota, Colombia, where predictive policing is being considered for deployment and a lot of public funds are invested. Publicly available data collected via surveys contains information on whether people were victims of a crime and whether they reported that crime at a district level. That allows you to see that the probability of someone reporting a crime where they were a victim varies across neighborhoods. We then showed that using this data to train predictive policing algorithms can lead to the misallocation of policing resources. If, for instance, the reporting rate in my district is half the reporting rate in your district, then my district needs to have twice as much crime for the system to observe the same amount of crime.
What sorts of problems can that cause?
There’s harm associated with misallocating the police — patrolling in a way that underpolices certain communities and overpolices certain communities. If your community has no access to police resources, that can lead to harm, and if your community is being overpoliced, especially if your community is often targeted by the police, that can also lead to harm.
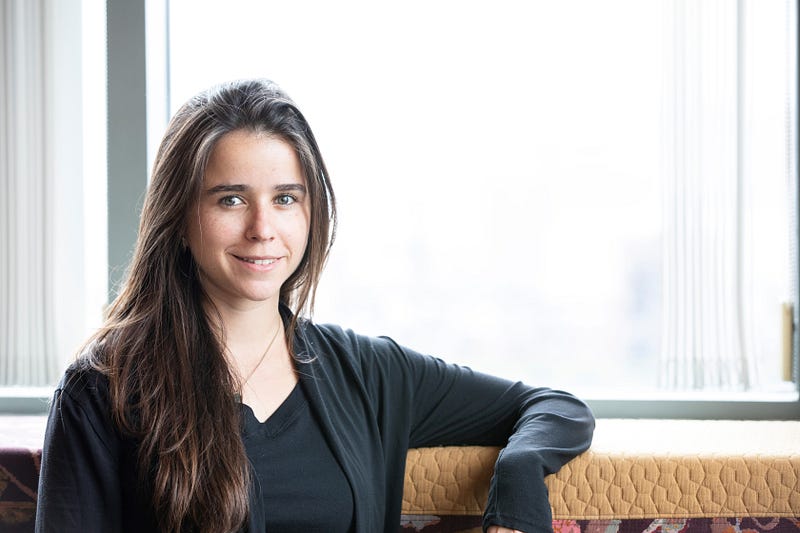
What other research areas are you interested in?
I’m interested in the collaboration between humans and algorithms. Algorithms make mistakes. I conducted an empirical study looking at an algorithm deployed in Allegheny County, Pennsylvania, to help child abuse hotline call workers determine whether a call that concerns potential child neglect or abuse requires investigation. They had a bug in their system when it was deployed, which led the system to misestimate certain scores. That allowed us to see how call workers react to misestimated scores. The findings were encouraging: Call workers did take the scores into consideration, but they were not blindly following the algorithm and instead they were more likely to overwrite erroneous recommendations.
Does that mean people and algorithms work well together?
Not always. Other research has found that is not necessarily the case. Sometimes humans can fall on the end of the spectrum we call automation bias, where they just blindly follow the algorithm. For example, “The algorithm is super smart. I should just trust anything it tells me.” On the opposite end, there’s algorithm aversion where you distrust everything the algorithm tells you regardless of its value: “This algorithm doesn’t know anything. It’s a machine. I obviously know better.” I’m interested in how we design and embed machine learning algorithms into a decision-making pipeline in a way that we’re augmenting the expert’s ability to make good decisions.
Story by Jeremy M. Simon
About this Post
Share: