Explainable AI
Global Analytics Summit examines efforts to understand how AI systems work and why that’s essential
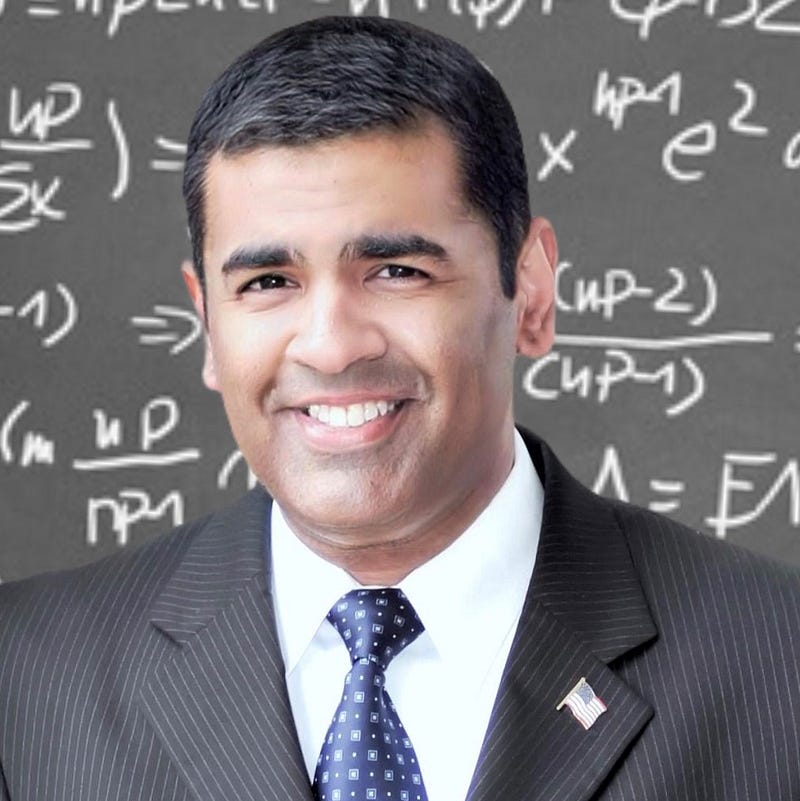
By Mark Barron
More than two dozen artificial intelligence experts from business and academia, including Texas McCombs, explored the importance of understanding how machine learning systems arrive at their conclusions so humans can trust those results.
This relatively new frontier of explainable AI, or XAI, was scrutinized for two half-day sessions in November during the online Global Analytics Summit held by the McCombs Center for Analytics and Transformative Technologies (CATT). More than 3,500 people registered for the conference, another step in the university’s drive to be a thought leader in the field.
Experts say that as AI systems become more sophisticated, they become increasingly difficult for humans to interpret. The calculation process turns into what is called a “black box” that even data scientists who create the algorithms can’t understand, according to IBM Corp. XAI methods, however, provide transparency so humans can understand the results and assess their accuracy and fairness.
“XAI is an important tool for business organizations and has a wide-ranging impact on major activities including risk management, compliance, ethics, reliability, and customer relationship management,” said Michael Sury, managing director of CATT.
Although AI is more than 50 years old, “deep learning has been a mini-scientific revolution” since the 2010s, said one keynote speaker, Charles Elkan, a professor of computer science at the University of California, San Diego. It has “enabled tasks that really look like they require remarkable intelligence because they require the combination of language and vision.” Elkan is also a former managing director for Goldman Sachs Group.
Black Boxes
A panel discussion called “Explainable vs. Ethical AI: Just Semantics?” followed Elkan’s presentation. The panelists sought to define some of the terms, themes, and paradigms of XAI, as well as examine the role of black boxes. Alex London, a professor of ethics and philosophy at Carnegie Mellon University, said that explainability and interpretability “express a relationship between the humans and the model that’s used in an AI system.”
Alice Xiang, a lawyer and a senior research scientist for Sony Group, said, “I see explainability as an important part of providing transparency and, in turn, enabling accountability.” She noted the challenge of black boxes, citing as examples drug-sniffing dogs, whose abilities are mysterious but highly accurate, and the horse Clever Hans, who appeared to understand math but was really following cues from its owner.
Polo Chau, an associate professor of computer science at Georgia Tech, pointed to the use of counterfactual tests — the turning on and off of parts of a model — as a way to test it. “It can be quite usable to a lot of users, including consumers,” he said.
Bias, Psychology, and Ethics
After that discussion, Krishnaram Kenthapadi, a principal scientist for Amazon Web Services AI, spoke on a panel entitled “Responsible AI in Industry” about how human bias can be reflected and amplified in the data. “There’s a risk we may be living in our own bubble and implementing some approaches where we may not be even aware of what may be the issues with these approaches,” he said.
In a panel discussion called “Adopting AI,” James Guszcza, a behavioral research affiliate at Stanford University and chief data scientist on leave from Deloitte LLP, said: “I think one of the previous speakers said we need to be interdisciplinary; I take it a little bit further and say we need to be transdisciplinary.” The work needs to involve machine learning and human psychology and ethics, he said. Without taking those into account, “You’re going to get artificial stupidity.”
Fellow panelist Anand Rao, the global AI lead for PwC (PricewaterhouseCoopers), noted that the human-computer interface is evolving and that society needs to be given time to adapt to new technology. “You need to be patient,” he said, referring especially to businesses.
And during his talk, Mark Johnson, chief AI scientist with Oracle Corp., said that humans are “at the very beginning” of learning how to use XAI to build better machine-learning models. He also said, “Data is the new oil,” noting that it is a valuable resource but comes in different grades, as do coal and crude, and needs to be processed and refined.
Different Approaches to XAI
McCombs assistant professor Maria De-Arteaga, who researches societal biases and machine learning, moderated a panel focused on XAI solutions.
Jette Henderson, a senior machine learning scientist with CognitiveScale Inc., said she works to help other companies understand their models. “So, I very much approach explainability from helping out customers.”
Zachary Lipton, an assistant professor of machine learning at Carnegie Mellon University, noted the disconnect between problems and solutions. Explainability is like chronic fatigue syndrome, he said. It’s an “all-encompassing bag … for a whole set of problems that don’t have a common solution.”
In talking about the mismatch between problems and solutions, panelist Scott Lundberg, a senior researcher at Microsoft Corp., said that the explanation could be actually hiding something about your model and its behavior.
One of the key risks of AI is a lack of transparency for clients, said Daniele Magazzeni, the AI research director with JPMorgan. Magazzeni said that his company has 80 research projects involving economic systems, data, ethics, crime, and more.
The final panel of the conference, moderated by Raymond Mooney, director of the UT AI Lab, took on the subject of “Explanations, But for Whom?”
Panelist Nazneen Rajani, a Salesforce research scientist, discussed how to evaluate explanations by giving them a score. Fellow panelist Christoforos Anagnostopoulos, senior data scientist at McKinsey & Co., pointed out the five principles of artificial intelligence in society, which were written about by Luciano Floridi and Josh Cowls, and which draw on the four principles of bioethics. The fifth AI principle is explainability, he said, and it is “probably the one thing that we should add … to have a complete framework of ethics.”
About this Post
Share: