New Research Tool Works When Control Groups Won’t
Where randomized experiments aren’t possible, researchers have a new statistical tool
Based on the research of Kathleen Li
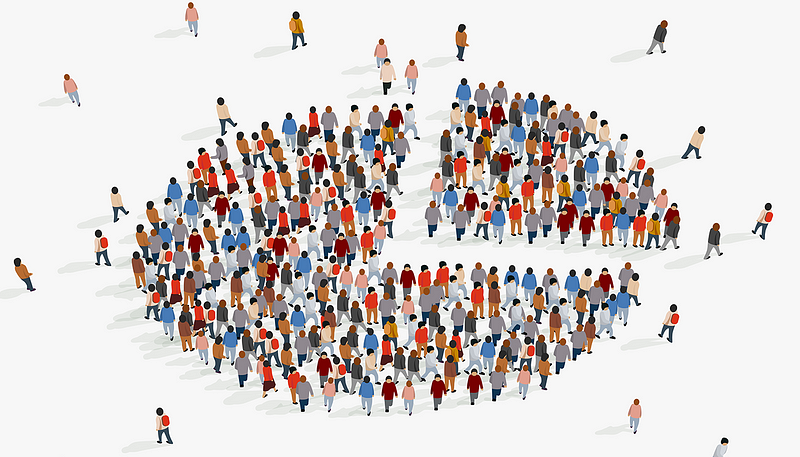
Whether they’re studying vaccine adoption rates or consumer preferences, randomized experiments are the gold standard in the world of research.
In such experiments, researchers split study participants into groups by chance. One group undergoes an intervention. The other — the control group — does not. Then, researchers can say with confidence whether a certain intervention made an impact.
In the real world, though, randomized experiments are not always possible, says Texas McCombs Assistant Professor of Marketing Kathleen Li. “In many situations, you simply can’t, because you can’t convince companies to do it, or maybe it’s against the law. It’s still important, however, to know an intervention’s effect.”
In new research, with Venkatesh Shankar of Texas A&M University, Li creates a statistical tool for such situations. Called two-step synthetic control, it can help researchers get meaningful results when randomized trials are not feasible.
“Our framework allows managers and policymakers to estimate effects they previously weren’t able to estimate accurately,” Li says. “They get a more precise estimate that can help them make more informed decisions.”
A More Flexible Approach
Li’s tool adapts an existing research workaround, known as the synthetic control method. As the name implies, it creates synthetic control groups from the data, in place of real ones. The groups are weighted statistically and compared with a group undergoing an intervention.
But the synthetic control method doesn’t perfectly apply to all situations, especially ones in which the intervention group is very different from its control groups. In these scenarios, the method could lead to less accurate results.
One problem, says Li, is that the method is somewhat inflexible. The control groups that make up its weighted combination must add up to 100%. For example, researchers could decide that one group accounts for 20% and another 80%.
A more flexible method might lead to more accurate results, the researchers say, and they’ve devised one. Their two-step synthetic control approach goes through two stages:
· First, it determines whether the traditional synthetic control method applies to a given case.
· If it does not, the second step uses a more flexible framework that allows weighted controls to differ from 100% or to shift the control group up and down.
“This approach balances the tradeoff,” Li says. “We first want to get an accurate effect, but at the same time, we also want to be as precise as possible in order to provide the most informative information to key decision makers.”
Accuracy, she adds, is about how close a measurement is to the true value. Precision, on the other hand, refers to the tightness of the numerical band the measurement is thought to fall in.
Measuring Tampon Sales
To test their new method on a real-world situation, Li and Shankar looked at sales of tampons: how they responded in 2016, when New York repealed a sales tax on them.
Sales taxes on tampons have been a contentious issue worldwide, and many countries — including Australia, Germany, and India — have abolished or reduced them. Proponents of repeal argue that feminine hygiene products are basic necessities and should not be taxed.
But by 2019, only 13 states in the U.S. had repealed the tax, with opponents arguing that repeal would decrease state revenues. One key point of contention for policymakers has been how repeal would affect tampon sales.
To find out, Li and Shankar gathered 52 weeks of sales data before New York’s repeal and 17 weeks after. Their control group was 35 states that did not repeal the tax.
In their first step, the researchers applied the traditional synthetic control method to the data. They found the traditional method probably overestimated the actual increase in weekly sales, showing a 2.5% rise in New York.
In the second step, the researchers applied a more flexible method. It estimated that New York’s repeal caused a more modest increase in weekly tampon sales, of only 2.08%. That estimate probably is more accurate, as the more flexible method matches the actual sales figures before the intervention better.
Any market or public policy researcher can use the new method, Li says. In fact, she and Shankar will be making it available online for all to use.
But she offers one caution: More flexible methods tend to be less precise, with wider bands of uncertainty.
“That’s the trade-off,” Li says. “You want to be able to use the method that’s just as flexible as you need it. It’s why having this tool to see how flexible you can go and still be precise is critical.”
“A Two-Step Synthetic Control Approach for Estimating Causal Effects of Marketing Events” is forthcoming, online in Management Science.
Story by Deborah Lynn Blumberg